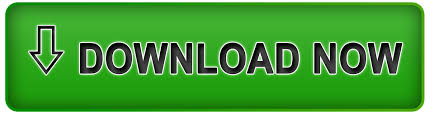
Applications are selected so as to avoid possible overlaps with the ones contained in this volume. Derivation of maximum entropy probability distribution for given fixed mean \(\mu\) and variance \(\sigma^\). This introductory chapter aims to spell out the basics of entropy, maximum entropy frameworks and their applications in some fields. The generalized maximum entropy estimator has played a vital role in the econometric model estimation because it is an alternative estimator to least squares (Wilawan Srichaikul, 2018).

Book Of Blogs, The Whalehead Club: Reflections Of Currituck HeritageSusan Joy Davis, Writings Of The Rev. Derivation of maximum entropy probability distribution with no other constraints (uniform distribution) The maximum entropy estimates the parameters in a linear regression model, especially when the data are ill-posed. Maximum Entropy, Information Without Probability And Complex Fractals: Classical And Quantum Approach (Fundamental Theories Of Physics) (Volume 112) Guy Jumarie, I Blog, Therefore I AmInc. While all probability models follow either Bayesian or Frequentist inference, they can yield vastly different results depending upon what specific parameter distribution algorithm is employed.Deriving probability distributions using the Principle of Maximum Entropy About posts ML Resources Deriving probability distributions using the Principle of Maximum Entropy Application of the maximum-entropy method to powder-diffraction data. However, under the maximum entropy principle, the researcher should go with whatever probability distribution they know the least about already.Ĭommon Probability Distribution Parameterizations in Machine Learning:
#MAXIMUM ENTROPY HOW TO#
They might be tempted to choose a probability function like Normal distribution, since knowing the mean first lets them fill in more variables in the prior assumption. We present a maximum-likelihood approach for automatically constructing maximum entropy models and describe how to implement this approach efficiently, using as.

statistical inference using the maximum likelihood method is used. For images with more than a million pixels, maximum entropy is faster than the CLEAN algorithm. Entropy measures such as Rnyi entropy, Havrda and Charvat entropy, Arimoto entropy. Maximum entropy is also called the all-poles model or autoregressive model. The goal is to maximize “uniformitiveness,” or uncertainty when making a prior probability assumption so that subjective bias is minimized in the model’s results.įor example, if only the mean of a certain parameter is known (the average outcome over long-term trials), then a researcher could use almost any probability distribution to build the model. A deconvolution algorithm (sometimes abbreviated MEM) which functions by minimizing a smoothness function (' entropy ') in an image. 2 What’s interesting about this latest work is that it studies dark matter interactions through entropy. Earlier studies 1 have hinted at this, and a new study supports the idea even further. It now seems clear that dark matter interacts more than just gravitationally. Using a communication-theory model for the process of image formation, we find that the most likely object has a maximum entropy and is represented by a. mass, energy, gravity, spin, and entropy define an exclusive presence of 1D Space.

The principle of maximum entropy is a model creation rule that requires selecting the most unpredictable (maximum entropy) prior assumption if only a single parameter is known about a probability distribution. The simulated distribution of dark matter in galaxies. with an end or thermal death at its maximum expansion (3D Space). Share edit What is the Principle of Maximum Entropy? Jayness principle of maximum entropy and Kullbacks principle of minimum cross-entropy (minimum directed divergence) are shown to be uniquely correct.
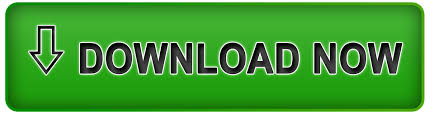